How is BodyScan able to capture a Users body fat percentage?
The field of estimating human body fat percentage is well-documented in existing published research, with nearly all reported methods relying on indirect measurements of adiposity, age, biological gender, and anthropometric measurements such as height, weight, BMI, waist circumference, and waist-hip ratio, as well as skin-fold thickness. While these indirect measures do have some correlation with body fat, they are 1-dimensional and offer limited degrees of freedom.
In contrast to existing approaches, our technology considers not only global features (e.g., biological sex, age, height, weight, BMI), but also additional distinguishing and specific local features (e.g., ratio of bone width, limb size) that we collect by directly, but confidentially, evaluating the outline of a person's body. To achieve this, we collated information from nearly 10,000 published research articles in the field of human body composition, including body fat. We then developed our patented and proprietary algorithms and validated this against several novel ideas using various protocols for data collection, processing, analysis, and modelling. Our estimated results are very accurate and useful to supply context to discussions between health professionals and users.
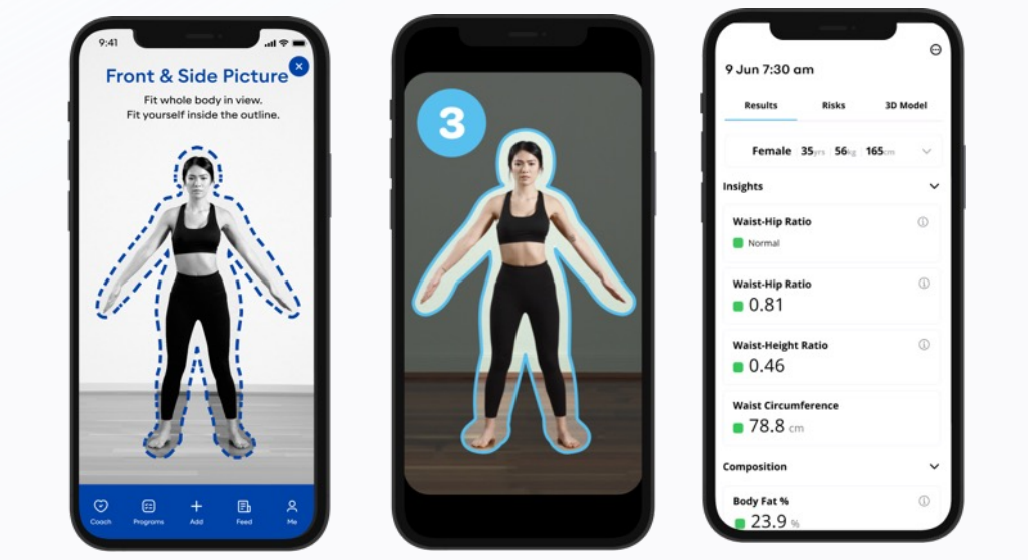
AHI conducted over 7,000 DEXA scans on individuals from different ethnicities. DEXA scans are medical-grade devices that provide a highly accurate measurement of body composition. In addition to the DEXA scans, we also took front and side photographs of each of the 7,000 participants. These 7,000 measurements were taken to act as an accurate reference point to validate the accuracy of AHI’s BodyScan.
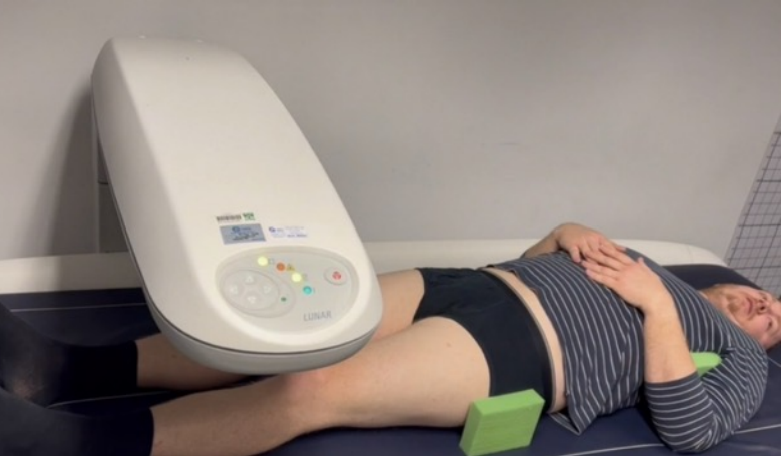
During each session, we collected photographs, DEXA scans, and other relevant data to ensure acceptable levels of accuracy and adhere to ethical and standardized data collection rules. All protocols were pre-designed and ethically and methodologically approved. We collaborated with research institutes and universities to ensure that these standards were met
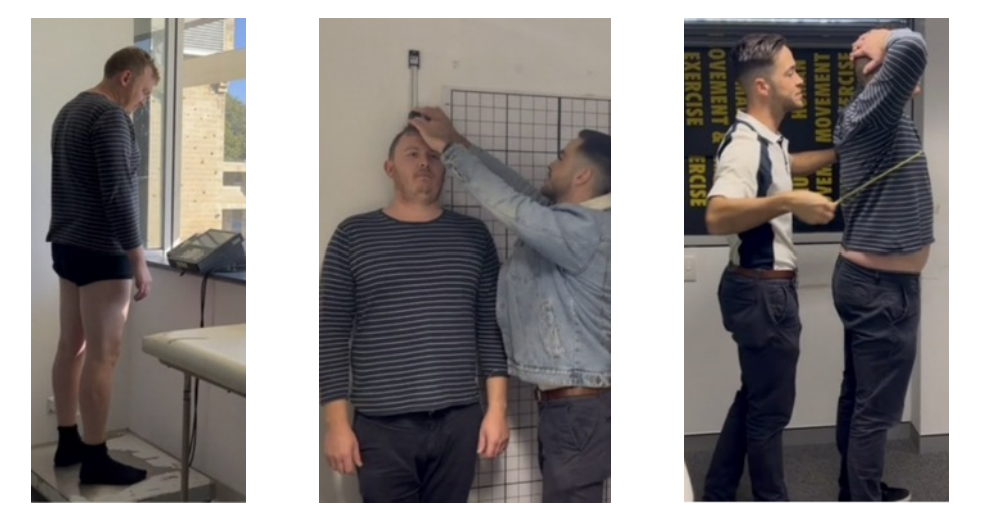
Following in depth statistical analysis and modelling of human data generated from various sources, we developed machine learning approaches that could learn and model the relationship between different human representations and features (both global and local). By doing so, we generated a corresponding 3D body shape and body composition parameter (body fat percentage). Unlike existing 1-dimensional models, our machine learning approaches can produce nonlinear transformations with many degrees of freedom, resulting in more accurate outputs.
Our AHI Lab process, called the “offline R&D phase,” involves the statistical analysis and modelling of the collected data. In an actual AHI BodyScan procedure, the user provides global features such as age, height, weight, biological gender, and ethnicity, and capture a front and side profile image. Our AI algorithm extracts and processes the relevant features, generating a 3D model of the user's body, which it then uses to predict other human body ratios and parameters, including body fat. To improve accuracy, reliability, and repeatability, we developed and validated our methods to filter noise from the captured images, remove outliers, and non-meaningful data. A confidentiality of the process is important, images are not stored.
Our reported accuracy ranges between 90-92.5% dependent on specific body shapes and ethnicities. However, the accuracy of our training models can increase to over 95% if a new client has a body shape and global features, similar to one or more of our existing data sets. Finally, the accuracy of our body fat measurements was published in journals with high repute, and was found to surpass the measurements obtained by expensive BIA (Bioelectrical Impedance Analysis) machines and was statistically equivalent to those derived from DEXA Scan.